4
0
0
0
0
Domain adaptation in computer vision applications
- 其他作者:
- 其他題名:
- Advances in computer vision and pattern recognition.
- 出版: Cham : Springer International Publishing :Imprint: Springer
- 叢書名: Advances in computer vision and pattern recognition,
- 主題: Computer vision. , Computer Science. , Image Processing and Computer Vision. , Artificial Intelligence (incl. Robotics) , Computer Appl. in Administrative Data Processing.
- ISBN: 9783319583471 (electronic bk.) 、 9783319583464 (paper)
-
FIND@SFXID:
CGU
- 資料類型: 電子書
- 內容註: A Comprehensive Survey on Domain Adaptation for Visual Applications -- A Deeper Look at Dataset Bias -- Part I: Shallow Domain Adaptation Methods -- Geodesic Flow Kernel and Landmarks: Kernel Methods for Unsupervised Domain Adaptation -- Unsupervised Domain Adaptation based on Subspace Alignment -- Learning Domain Invariant Embeddings by Matching Distributions -- Adaptive Transductive Transfer Machines: A Pipeline for Unsupervised Domain Adaptation -- What To Do When the Access to the Source Data is Constrained? -- Part II: Deep Domain Adaptation Methods -- Correlation Alignment for Unsupervised Domain Adaptation -- Simultaneous Deep Transfer Across Domains and Tasks -- Domain-Adversarial Training of Neural Networks -- Part III: Beyond Image Classification -- Unsupervised Fisher Vector Adaptation for Re-Identification -- Semantic Segmentation of Urban Scenes via Domain Adaptation of SYNTHIA -- From Virtual to Real World Visual Perception using Domain Adaptation - The DPM as Example -- Generalizing Semantic Part Detectors Across Domains -- Part IV: Beyond Domain Adaptation: Unifying Perspectives -- A Multi-Source Domain Generalization Approach to Visual Attribute Detection -- Unifying Multi-Domain Multi-Task Learning: Tensor and Neural Network Perspectives.
- 摘要註: This comprehensive text/reference presents a broad review of diverse domain adaptation (DA) methods for machine learning, with a focus on solutions for visual applications. The book collects together solutions and perspectives proposed by an international selection of pre-eminent experts in the field, addressing not only classical image categorization, but also other computer vision tasks such as detection, segmentation and visual attributes. Topics and features: Surveys the complete field of visual DA, including shallow methods designed for homogeneous and heterogeneous data as well as deep architectures Presents a positioning of the dataset bias in the CNN-based feature arena Proposes detailed analyses of popular shallow methods that addresses landmark data selection, kernel embedding, feature alignment, joint feature transformation and classifier adaptation, or the case of limited access to the source data Discusses more recent deep DA methods, including discrepancy-based adaptation networks and adversarial discriminative DA models Addresses domain adaptation problems beyond image categorization, such as a Fisher encoding adaptation for vehicle re-identification, semantic segmentation and detection trained on synthetic images, and domain generalization for semantic part detection Describes a multi-source domain generalization technique for visual attributes and a unifying framework for multi-domain and multi-task learning This authoritative volume will be of great interest to a broad audience ranging from researchers and practitioners, to students involved in computer vision, pattern recognition and machine learning. Dr. Gabriela Csurka is a Senior Scientist in the Computer Vision Team at Xerox Research Centre Europe, Meylan, France.
-
讀者標籤:
- 系統號: 005408456 | 機讀編目格式
館藏資訊
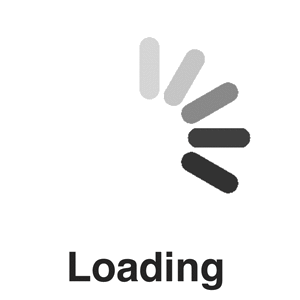