1
0
0
0
0
Machine learning in medicine a complete overview / [electronic resource] :
- 作者: Cleophas, Ton J.
- 其他作者:
- 出版: Cham : Springer International Publishing :Imprint: Springer
- 主題: Machine learning , Medical informatics , Artificial intelligence--Medical applications , Biomedicine. , Biomedicine general. , Medicine/Public Health, general. , Statistics, general. , Science, general.
- ISBN: 9783319151953 (electronic bk.) 、 9783319151946 (paper)
-
FIND@SFXID:
CGU
- 資料類型: 電子書
-
課程:
智慧醫療工程 ( 人工智慧碩士班 - 陳冠甫)
智慧醫療工程 ( 臨醫所博班人工智慧組 - 陳冠甫) - 內容註: Preface. Section I Cluster and Classification Models -- Hierarchical Clustering and K-means Clustering to Identify Subgroups in Surveys (50 Patients) -- Density-based Clustering to Identify Outlier Groups in Otherwise Homogeneous Data (50 Patients) -- Two Step Clustering to Identify Subgroups and Predict Subgroup Memberships in Individual Future Patients (120 Patients)- Nearest Neighbors for Classifying New Medicines (2 New and 25 Old Opioids)- Predicting High-Risk-Bin Memberships (1445 Families) -- Predicting Outlier Memberships (2000 Patients) -- Data Mining for Visualization of Health Processes (150 Patients) -- 8 Trained Decision Trees for a More Meaningful Accuracy (150 Patients) -- Typology of Medical Data (51 Patients) -- Predictions from Nominal Clinical Data (450 Patients) -- Predictions from Ordinal Clinical Data (450 Patients) -- Assessing Relative Health Risks (3000 Subjects) -- Measurement Agreements (30 Patients) -- Column Proportions for Testing Differences between Outcome Scores (450 Patients) -- Pivoting Trays and Tables for Improved Analysis of Multidimensional Data (450 Patients) -- Online Analytical Procedure Cubes for a More Rapid Approach to Analyzing Frequencies (450 Patients) -- Restructure Data Wizard for Data Classified the Wrong Way (20 Patients) -- Control Charts for Quality Control of Medicines (164 Tablet Desintegration Times) -- Section II (Log) Linear Models -- Linear, Logistic, and Cox Regression for Outcome Prediction with Unpaired Data (20, 55, and 60 Patients) -- Generalized Linear Models for Outcome Prediction with Paired Data (100 Patients and 139 Physicians) -- Generalized Linear Models for Predicting Event-Rates (50 Patients) -- Factor Analysis and Partial Least Squares (PLS) for Complex-Data Reduction (250 Patients) -- Optimal Scaling of High-sensitivity Analysis of Health Predictors (250 Patients) -- Discriminant Analysis for Making a Diagnosis from Multiple Outcomes (45 Patients) -- Weighted Least Squares for Adjus
- 摘要註: The current book is the first publication of a complete overview of machine learning methodologies for the medical and health sector. It was written as a training companion, and as a must-read, not only for physicians and students, but also for any one involved in the process and progress of health and health care. In eighty chapters eighty different machine learning methodologies are reviewed, in combination with data examples for self-assessment. Each chapter can be studied without the need to consult other chapters. The amount of data stored in the world's databases doubles every 20 months, and clinicians, familiar with traditional statistical methods, are at a loss to analyze them. Traditional methods have, indeed, difficulty to identify outliers in large datasets, and to find patterns in big data and data with multiple exposure / outcome variables. In addition, analysis-rules for surveys and questionnaires, which are currently common methods of data collection, are, essentially, missing. Fortunately, the new discipline, machine learning, is able to cover all of these limitations. So far medical professionals have been rather reluctant to use machine learning. Also, in the field of diagnosis making, few doctors may want a computer checking them, are interested in collaboration with a computer or with computer engineers. Adequate health and health care will, however, soon be impossible without proper data supervision from modern machine learning methodologies like cluster models, neural networks, and other data mining methodologies. Each chapter starts with purposes and scientific questions. Then, step-by-step analyses, using data examples, are given. Finally, a paragraph with conclusion, and references to the corresponding sites of three introductory textbooks, previously written by the same authors, is given.
-
讀者標籤:
- 系統號: 005132544 | 機讀編目格式
館藏資訊
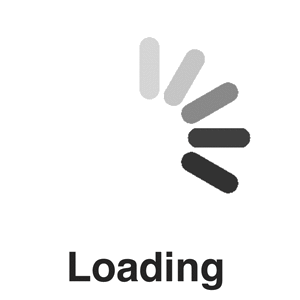