Machine learning for evolution strategies
- 作者: Kramer, Oliver, author.
- 其他作者:
- 其他題名:
- Studies in big data ;
- 出版: Cham : Springer International Publishing :Imprint: Springer
- 叢書名: Studies in big data,volume 20
- 主題: Machine learning. , Engineering. , Computational Intelligence. , Simulation and Modeling. , Data Mining and Knowledge Discovery. , Socio- and Econophysics, Population and Evolutionary Models. , Artificial Intelligence (incl. Robotics)
- ISBN: 9783319333830 (electronic bk.) 、 9783319333816 (paper)
-
FIND@SFXID:
CGU
- 資料類型: 電子書
- 內容註: Part I Evolution Strategies -- Part II Machine Learning -- Part III Supervised Learning.
- 摘要註: This book introduces numerous algorithmic hybridizations between both worlds that show how machine learning can improve and support evolution strategies. The set of methods comprises covariance matrix estimation, meta-modeling of fitness and constraint functions, dimensionality reduction for search and visualization of high-dimensional optimization processes, and clustering-based niching. After giving an introduction to evolution strategies and machine learning, the book builds the bridge between both worlds with an algorithmic and experimental perspective. Experiments mostly employ a (1+1)-ES and are implemented in Python using the machine learning library scikit-learn. The examples are conducted on typical benchmark problems illustrating algorithmic concepts and their experimental behavior. The book closes with a discussion of related lines of research.
-
讀者標籤:
- 系統號: 005361345 | 機讀編目格式
館藏資訊
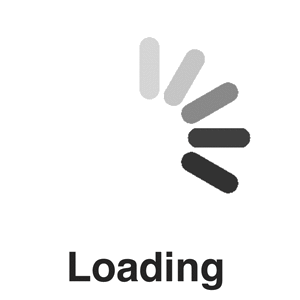
This book introduces numerous algorithmic hybridizations between both worlds that show how machine learning can improve and support evolution strategies. The set of methods comprises covariance matrix estimation, meta-modeling of fitness and constraint functions, dimensionality reduction for search and visualization of high-dimensional optimization processes, and clustering-based niching. After giving an introduction to evolution strategies and machine learning, the book builds the bridge between both worlds with an algorithmic and experimental perspective. Experiments mostly employ a (1+1)-ES and are implemented in Python using the machine learning library scikit-learn. The examples are conducted on typical benchmark problems illustrating algorithmic concepts and their experimental behavior. The book closes with a discussion of related lines of research.