Stochastic parameterizing manifolds and non-markovian reduced equations Stochastic Manifolds for Nonlinear SPDEs II / [electronic resource] :
- 作者: Chekroun, Mickael D.
- 其他作者:
- 其他題名:
- SpringerBriefs in mathematics,
- 出版: Cham : Springer International Publishing :Imprint: Springer
- 叢書名: SpringerBriefs in mathematics,
- 主題: Stochastic partial differential equations--Numerical solutions. , Mathematics , Partial Differential Equations. , Dynamical Systems and Ergodic Theory. , Probability Theory and Stochastic Processes. , Ordinary Differential Equations.
- ISBN: 9783319125206 (electronic bk.) 、 9783319125190 (paper)
-
FIND@SFXID:
CGU
- 資料類型: 電子書
- 內容註: General Introduction -- Preliminaries -- Invariant Manifolds -- Pullback Characterization of Approximating, and Parameterizing Manifolds -- Non-Markovian Stochastic Reduced Equations -- On-Markovian Stochastic Reduced Equations on the Fly -- Proof of Lemma 5.1.-References -- Index.
- 摘要註: In this second volume, a general approach is developed to provide approximate parameterizations of the "small" scales by the "large" ones for a broad class of stochastic partial differential equations (SPDEs). This is accomplished via the concept of parameterizing manifolds (PMs), which are stochastic manifolds that improve, for a given realization of the noise, in mean square error the partial knowledge of the full SPDE solution when compared to its projection onto some resolved modes. Backward-forward systems are designed to give access to such PMs in practice. The key idea consists of representing the modes with high wave numbers as a pullback limit depending on the time-history of the modes with low wave numbers. Non-Markovian stochastic reduced systems are then derived based on such a PM approach. The reduced systems take the form of stochastic differential equations involving random coefficients that convey memory effects. The theory is illustrated on a stochastic Burgers-type equation.
-
讀者標籤:
- 系統號: 005127399 | 機讀編目格式
館藏資訊
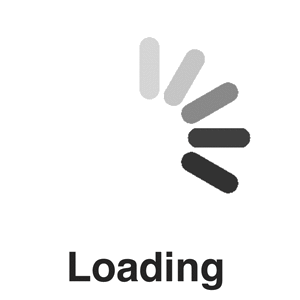
In this second volume, a general approach is developed to provide approximate parameterizations of the "small" scales by the "large" ones for a broad class of stochastic partial differential equations (SPDEs). This is accomplished via the concept of parameterizing manifolds (PMs), which are stochastic manifolds that improve, for a given realization of the noise, in mean square error the partial knowledge of the full SPDE solution when compared to its projection onto some resolved modes. Backward-forward systems are designed to give access to such PMs in practice. The key idea consists of representing the modes with high wave numbers as a pullback limit depending on the time-history of the modes with low wave numbers. Non-Markovian stochastic reduced systems are then derived based on such a PM approach. The reduced systems take the form of stochastic differential equations involving random coefficients that convey memory effects. The theory is illustrated on a stochastic Burgers-type equation.