Machine learning with quantum computers [electronic resource] :
- 作者: Schuld, Maria, author.
- 其他作者:
- 其他題名:
- Quantum science and technology.
- 出版: Cham : Springer International Publishing :Imprint: Springer
- 版本:Second edition.
- 叢書名: Quantum science and technology,
- 主題: Machine learning. , Quantum computing. , Quantum Computing. , Machine Learning. , Mathematics, general.
- ISBN: 9783030830984 (electronic bk.) 、 9783030830977 (paper)
-
FIND@SFXID:
CGU
- 資料類型: 電子書
- 課程: 量子機器學習(英語授課) ( 人工智慧學系 - RENATA WONG)
- 內容註: Chapter 1. Introduction -- Chapter 2. Machine Learning -- Chapter 3. Quantum Computing -- Chapter 4. Representing Data on a Quantum Computer -- Chapter 5. Variational Circuits as Machine Learning Models -- Chapter 6. Quantum Models as Kernel Methods -- Chapter 7. Fault-Tolerant Quantum Machine Learning -- Chapter 8. Approaches Based on the Ising Model -- Chapter 9. Potential Quantum Advantages.
- 摘要註: This book offers an introduction into quantum machine learning research, covering approaches that range from "near-term" to fault-tolerant quantum machine learning algorithms, and from theoretical to practical techniques that help us understand how quantum computers can learn from data. Among the topics discussed are parameterized quantum circuits, hybrid optimization, data encoding, quantum feature maps and kernel methods, quantum learning theory, as well as quantum neural networks. The book aims at an audience of computer scientists and physicists at the graduate level onwards. The second edition extends the material beyond supervised learning and puts a special focus on the developments in near-term quantum machine learning seen over the past few years.
-
讀者標籤:
- 系統號: 005529211 | 機讀編目格式
館藏資訊
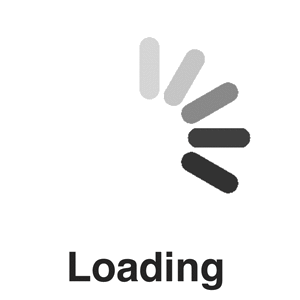
This book offers an introduction into quantum machine learning research, covering approaches that range from "near-term" to fault-tolerant quantum machine learning algorithms, and from theoretical to practical techniques that help us understand how quantum computers can learn from data. Among the topics discussed are parameterized quantum circuits, hybrid optimization, data encoding, quantum feature maps and kernel methods, quantum learning theory, as well as quantum neural networks. The book aims at an audience of computer scientists and physicists at the graduate level onwards. The second edition extends the material beyond supervised learning and puts a special focus on the developments in near-term quantum machine learning seen over the past few years.