Proceedings of ELM2019
- 作者: International Conference on Extreme Learning Machine (2019 : Yangzhou Shi, China)
- 其他作者:
- 其他題名:
- ELM2019
- Proceedings in adaptation, learning and optimization ;
- 出版: Cham : Springer International Publishing :Imprint: Springer
- 叢書名: Proceedings in adaptation, learning and optimization,volume 14
- 主題: Artificial intelligence--Congresses. , Computational intelligence--Congresses. , Computational Intelligence. , Artificial Intelligence.
- ISBN: 9783030589899 (electronic bk.) 、 9783030589882 (paper)
-
FIND@SFXID:
CGU
- 資料類型: 電子書
- 內容註: Evolutionary Extreme Learning Machine Based Weighted Fuzzy-rough Nearest-neighbour Algorithm -- NNRW-based algorithm selection for software model checking -- An extreme learning machine method for diagnosis of patellofemoral pain syndrome -- Extreme Learning Machines for Signature Verification -- Website Classification from Webpage Renders -- ELM Algorithms Optimized by WOA for Motor Imagery Classification -- The Octonion Extreme Learning Machine -- Scikit-ELM: an Extreme Learning Machine toolbox for dynamic and scalable learning -- High-performance ELM for Memory Constrained Edge Computing Devices with Metal Performance Shaders -- Validating Untrained Human Annotations using Extreme Learning Machines -- ELM Feature Selection and SOM Data Visualization for Nursing Survey Dataset.
- 摘要註: This book contains some selected papers from the International Conference on Extreme Learning Machine 2019, which was held in Yangzhou, China, December 14-16, 2019. Extreme Learning Machines (ELMs) aim to enable pervasive learning and pervasive intelligence. As advocated by ELM theories, it is exciting to see the convergence of machine learning and biological learning from the long-term point of view. ELM may be one of the fundamental 'learning particles' filling the gaps between machine learning and biological learning (of which activation functions are even unknown) ELM represents a suite of (machine and biological) learning techniques in which hidden neurons need not be tuned: inherited from their ancestors or randomly generated. ELM learning theories show that effective learning algorithms can be derived based on randomly generated hidden neurons (biological neurons, artificial neurons, wavelets, Fourier series, etc) as long as they are nonlinear piecewise continuous, independent of training data and application environments. Increasingly, evidence from neuroscience suggests that similar principles apply in biological learning systems. ELM theories and algorithms argue that "random hidden neurons" capture an essential aspect of biological learning mechanisms as well as the intuitive sense that the efficiency of biological learning need not rely on computing power of neurons. ELM theories thus hint at possible reasons why the brain is more intelligent and effective than current computers. The main theme of ELM2019 is Hierarchical ELM, AI for IoT, Synergy of Machine Learning and Biological Learning. This conference provides a forum for academics, researchers and engineers to share and exchange R&D experience on both theoretical studies and practical applications of the ELM technique and brain learning. This book covers theories, algorithms and applications of ELM. It gives readers a glance of the most recent advances of ELM.
-
讀者標籤:
- 系統號: 005494387 | 機讀編目格式
館藏資訊
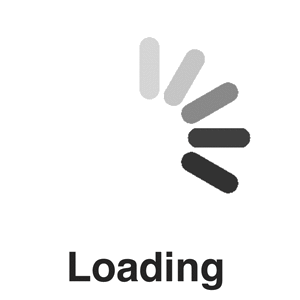
This book contains some selected papers from the International Conference on Extreme Learning Machine 2019, which was held in Yangzhou, China, December 14–16, 2019. Extreme Learning Machines (ELMs) aim to enable pervasive learning and pervasive intelligence. As advocated by ELM theories, it is exciting to see the convergence of machine learning and biological learning from the long-term point of view. ELM may be one of the fundamental ‘learning particles’ filling the gaps between machine learning and biological learning (of which activation functions are even unknown). ELM represents a suite of (machine and biological) learning techniques in which hidden neurons need not be tuned: inherited from their ancestors or randomly generated. ELM learning theories show that effective learning algorithms can be derived based on randomly generated hidden neurons (biological neurons, artificial neurons, wavelets, Fourier series, etc) as long as they are nonlinear piecewise continuous, independent of training data and application environments. Increasingly, evidence from neuroscience suggests that similar principles apply in biological learning systems. ELM theories and algorithms argue that “random hidden neurons” capture an essential aspect of biological learning mechanisms as well as the intuitive sense that the efficiency of biological learning need not rely on computing power of neurons. ELM theories thus hint at possible reasons why the brain is more intelligent and effective than current computers. The main theme of ELM2019 is Hierarchical ELM, AI for IoT, Synergy of Machine Learning and Biological Learning. This conference provides a forum for academics, researchers and engineers to share and exchange R&D experience on both theoretical studies and practical applications of the ELM technique and brain learning. This book covers theories, algorithms and applications of ELM. It gives readers a glance of the most recent advances of ELM.