8
0
0
0
0
Artificial intelligence : a modern approach
- 作者: Russell, Stuart J. (Stuart Jonathan)
- 其他作者:
- 其他題名:
- Prentice Hall series in artificial intelligence
- 出版: Upper Saddle River, NJ : Prentice Hall
- 版本:3rd ed.
- 叢書名: Prentice Hall series in artificial intelligence
- 主題: Artificial intelligence , Artificial intelligence
- ISBN: 9780136042594 (hbk.): US$155.38 、 0136042597 、 9780132071482 (pbk.) 、 0132071487 (pbk.)
- 資料類型: 圖書
- 課程: 人工智慧及機器學習導論 ( 人工智慧碩士班 - 蘇豐文)
- 內容註: Includes bibliographical references (pages 1063-1093) and index. Artificial Intelligence: -- Introduction: -- What is AI? -- Foundations of artificial intelligence -- History of artificial intelligence -- State of the art -- Summary, bibliographical and historical notes, exercises -- Intelligent agents: -- Agents and environments -- Good behavior: concept of rationality -- Nature of environments -- Structure of agents -- Summary, bibliographical and historical notes, exercises -- Problem-Solving: -- Solving problems by searching: -- Problem-solving agents -- Example problems -- Searching for solutions -- Uniformed search strategies -- Informed (heuristic) search strategies -- Heuristic functions -- Summary, bibliographical and historical notes, exercises -- Beyond classical search: -- Local search algorithms and optimization problems -- Local search in continuous spaces -- Searching with nondeterministic actions -- Searching with partial observations -- Online search agents and unknown environments -- Summary, bibliographical and historical notes, exercises -- Adversarial search: -- Games -- Optimal decisions in games -- Alpha-beta pruning -- Imperfect real-time decisions -- Stochastic games -- Partially observable games -- State-of-the-art game programs -- Alternative approaches -- Summary, bibliographical and historical notes, exercises -- Constraint satisfaction problems: -- Defining constraint satisfaction problems -- Constraint propagation: inference in CSPs -- Backtracking search for CSPs -- Local search for CSPs -- Structure of problems -- Summary, bibliographical and historical notes, exercises -- Knowledge, Reasoning, And Planning: -- Logical agents: -- Knowledge-based agents -- Wumpus world -- Logic -- Propositional logic: a very simple logic -- Propositional theorem proving -- Effective propositional model checking -- Agents based on propositional logic -- Summary, bibliographical and historical notes, exercises -- First-order logic: -- Representation revisited -- Syntax and semantics of first-order logic -- Usi Uncertain Knowledge And Reasoning: -- Quantifying uncertainty: -- Acting under uncertainty -- Basic probability notation -- Inference using full joint distributions -- Independence -- Bayes' rule and its use -- Wumpus world revisited -- Summary, bibliographical and historical notes, exercises -- Probabilistic reasoning: -- Representing knowledge in an uncertain domain -- Semantics of Bayesian networks -- Efficient representation of conditional distributions -- Exact inference in Bayesian networks -- Approximate inference in Bayesian networks -- Relational and first-order probability models -- Other approaches to uncertain reasoning -- Summary, bibliographical and historical notes, exercises -- Probabilistic reasoning over time: -- Time an uncertainty -- Inference in temporal models -- Hidden markov models -- Kalman filters -- Dynamic Bayesian networks -- Keeping track of many objects -- Summary, bibliographical and historical notes, exercises -- Making simple decisions: -- Combining beliefs and desires under uncertainty -- Basis of utility theory -- Utility functions -- Multiattribute utility functions -- Decision networks -- Value of information -- Decision-theoretic expert systems -- Summary, bibliographical and historical notes, exercises -- Making complex decisions: -- Sequential decision problems -- Value iteration -- Policy iteration -- Partially observable MDPs -- Decisions with multiple agents: game theory -- Mechanism design -- Summary, bibliographical and historical notes, exercises -- Learning: -- Learning from examples: -- Forms of learning -- Supervised learning -- Learning decision trees -- Evaluating and choosing the best hypothesis -- Theory of learning -- Regression and classification with linear models -- Artificial neural networks -- Nonparametric models -- Support vector machines -- Ensemble learning -- Practical machine learning -- Summary, bibliographical and historical notes, exercises -- Knowledge in learning: -- Logical formulation of
- 摘要註: In this third edition, the authors have updated the treatment of all major areas. A new organizing principle--the representational dimension of atomic, factored, and structured models--has been added. Significant new material has been provided in areas such as partially observable search, contingency planning, hierarchical planning, relational and first-order probability models, regularization and loss functions in machine learning, kernel methods, Web search engines, information extraction, and learning in vision and robotics. The book also includes hundreds of new exercises.
-
讀者標籤:
- 系統號: 005123918 | 機讀編目格式
館藏資訊
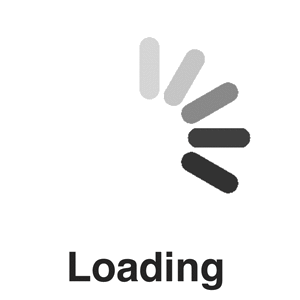
Artificial intelligence: A Modern Approach, 3e,is ideal for one or two-semester, undergraduate or graduate-level courses in Artificial Intelligence. It is also a valuable resource for computer professionals, linguists, and cognitive scientists interested in artificial intelligence. The revision of this best-selling text offers the most comprehensive, up-to-date introduction to the theory and practice of artificial intelligence.
資料來源:
Google Book
延伸查詢
Google Books
Amazon