Visual and text sentiment analysis through hierarchical deep learning networks
- 作者: Chaudhuri, Arindam, author.
- 其他作者:
- 其他題名:
- SpringerBriefs in computer science.
- 出版: Singapore : Springer Singapore :Imprint: Springer
- 叢書名: SpringerBriefs in computer science,
- 主題: Natural language processing (Computer science) , Computational linguistics. , Public opinion--Data processing. , Data mining. , Information Storage and Retrieval. , Database Management. , Data Mining and Knowledge Discovery. , Pattern Recognition.
- ISBN: 9789811374746 (electronic bk.) 、 9789811374739 (paper)
-
FIND@SFXID:
CGU
- 資料類型: 電子書
- 內容註: Chapter1. Introduction -- Chapter 2. Current State of Art -- Chapter 3. Literature Review -- Chapter 4. Twitter Datasets Used -- Chapter 5. Visual and Text Sentiment Analysis -- Chapter 6. Experimental Setup: Visual and Text Sentiment Analysis through Hierarchical Deep Learning Networks -- Chapter 7. Twitter Datasets Used -- Chapter 8. Experimental Results -- Chapter 9. Conclusion.
- 摘要註: This book presents the latest research on hierarchical deep learning for multi-modal sentiment analysis. Further, it analyses sentiments in Twitter blogs from both textual and visual content using hierarchical deep learning networks: hierarchical gated feedback recurrent neural networks (HGFRNNs) Several studies on deep learning have been conducted to date, but most of the current methods focus on either only textual content, or only visual content. In contrast, the proposed sentiment analysis model can be applied to any social blog dataset, making the book highly beneficial for postgraduate students and researchers in deep learning and sentiment analysis. The mathematical abstraction of the sentiment analysis model is presented in a very lucid manner. The complete sentiments are analysed by combining text and visual prediction results. The book's novelty lies in its development of innovative hierarchical recurrent neural networks for analysing sentiments; stacking of multiple recurrent layers by controlling the signal flow from upper recurrent layers to lower layers through a global gating unit; evaluation of HGFRNNs with different types of recurrent units; and adaptive assignment of HGFRNN layers to different timescales. Considering the need to leverage large-scale social multimedia content for sentiment analysis, both state-of-the-art visual and textual sentiment analysis techniques are used for joint visual-textual sentiment analysis. The proposed method yields promising results from Twitter datasets that include both texts and images, which support the theoretical hypothesis.
-
讀者標籤:
- 系統號: 005450175 | 機讀編目格式
館藏資訊
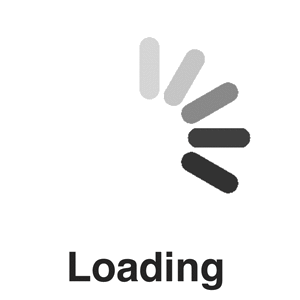
This book presents the latest research on hierarchical deep learning for multi-modal sentiment analysis. Further, it analyses sentiments in Twitter blogs from both textual and visual content using hierarchical deep learning networks: hierarchical gated feedback recurrent neural networks (HGFRNNs). Several studies on deep learning have been conducted to date, but most of the current methods focus on either only textual content, or only visual content. In contrast, the proposed sentiment analysis model can be applied to any social blog dataset, making the book highly beneficial for postgraduate students and researchers in deep learning and sentiment analysis. The mathematical abstraction of the sentiment analysis model is presented in a very lucid manner. The complete sentiments are analysed by combining text and visual prediction results. The book’s novelty lies in its development of innovative hierarchical recurrent neural networks for analysing sentiments; stacking of multiple recurrent layers by controlling the signal flow from upper recurrent layers to lower layers through a global gating unit; evaluation of HGFRNNs with different types of recurrent units; and adaptive assignment of HGFRNN layers to different timescales. Considering the need to leverage large-scale social multimedia content for sentiment analysis, both state-of-the-art visual and textual sentiment analysis techniques are used for joint visual-textual sentiment analysis. The proposed method yields promising results from Twitter datasets that include both texts and images, which support the theoretical hypothesis.